Introduction
The year 2020 was challenging for the entire world, everyone in the world had one thing in common. We all struggled with carrying about our day-to-day activities - like office, college, school, shopping, etc. - sitting at home! It changed our life as we know it.
With such an impact on an individual level, just imagine what many organisations might have had to face. They had to digitise everything - in a very short time without any planning. No business trips or conferences or meetings! But it also led to many benefits like being able to attend a conference halfway across the world, without even moving from your house!
Now with this digitisation comes a great deal of data and people have started realising how important this data is - and we aren't just talking about the internal data, we are talking about the external data - the one which comes from your partners, suppliers, customers, etc. We have so much data and now we can exploit this data using Machine Learning (ML) and Artificial Intelligence (AI).
What is external data, and why does it matter?
External data is the data that's been captured, processed, and provided from outside of your business.

This data is critical to ensure that you make the right business decisions by seeing the big picture - which is something you cannot have simply from your own organisation's internal data.
How to use this external data?
External data is enormous and we need the ability to scan through zettabytes (ZBs) of data within minutes - which is possible only using ML/AI algorithms. Previously, organizations that could tweak their algorithms had an edge but that's no more the case. With algorithms becoming open source, development environments like Azure Synapse, Delta Lake and Snowpark from Snowflake allow everybody easy access to ML algorithms and the ML environment. You don't have to be a hardcore data scientist anymore, it's getting easier for even small businesses to get into this and compete better.
Data Privacy and Security:

With a great amount of data comes the responsibility of securing it well enough from potential fraud and breach both internally and externally. Identifying who has access to which data and making sure its appropriate is getting increasingly important. Regulations have now started to get into this area and have started demanding better handling of data - General Data Protection Regulation (GDPR) is one such example.

To work securely with somebody else's data which is external data for you - we can have a practice where one can provide the data, the other can work on it and the other can consume it - this is something that can emerge now.
Below is the summary of a recent Gartner report which explains barriers to AI implementation - we can see that some top barriers are data related. It is crucial to make sure that external data is handled properly to make any AI implementation successful.
Explorium surveyed data leaders from across industries around what they feel about external data - Most say that they find external data very valuable but at the same time struggle to find the data they need.
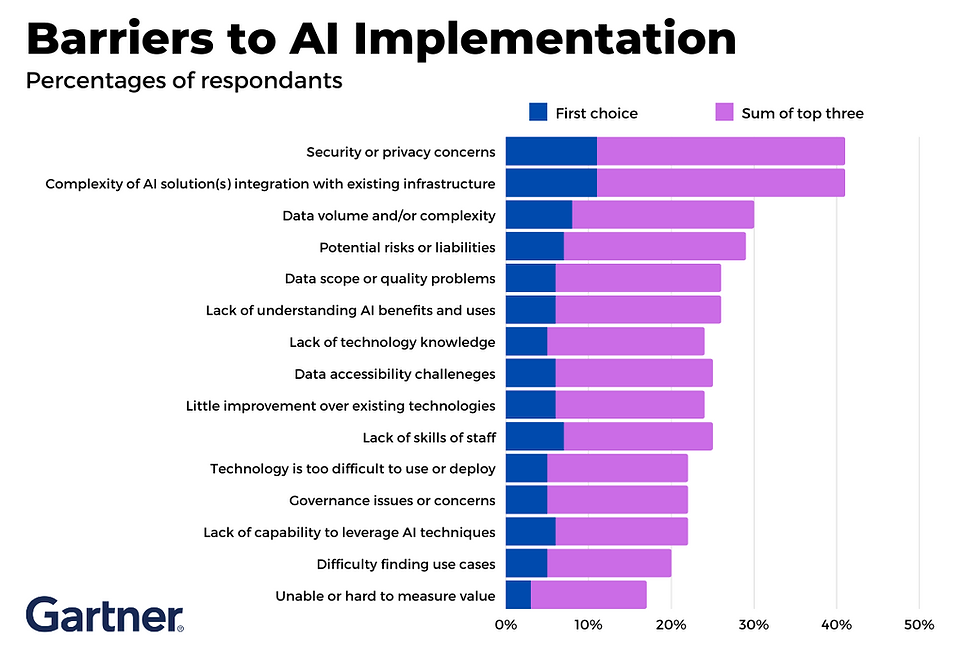
Third Party Data - What is it?
This data is any information collected by an entity that does not have a direct relationship with the user that the data is being collected on. This can be the data from your partners, suppliers, and customers which you should be using.
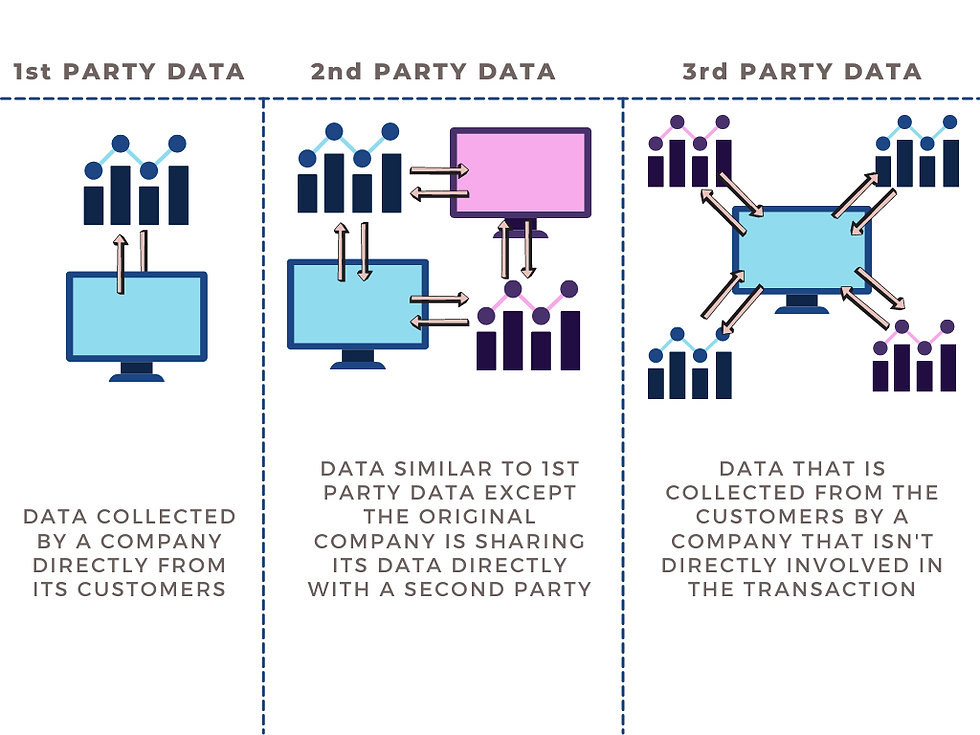
A company or brand can go beyond its usual audience using third-party data. This lets companies grow the size of their targeted audience. For example, new prospects who buy similar or complementary products or services from a direct competitor or a partner company can be included. Example of using third party data: Weather data being used to study the impact on the foot traffic in a store. In terms of data, what always matters is the richness and not the volume.
Challenges:
Since we have to collect external data from various data sources, it's almost always in different forms and sizes where we can have APIs for some of those, for some we can have data sets that need to be pulled in.

It thus becomes vital that we have the tools to be able to pull those diverse types of datasets. With the use of the cloud, we can diminish the effort of downloading the data needlessly.
Until recently, the data acquisition process was -
Finding the relevant data
Buy the data
Integrate it
Match it
Monitor and maintain the data on an ongoing basis - If it is relevant to what you are trying to achieve
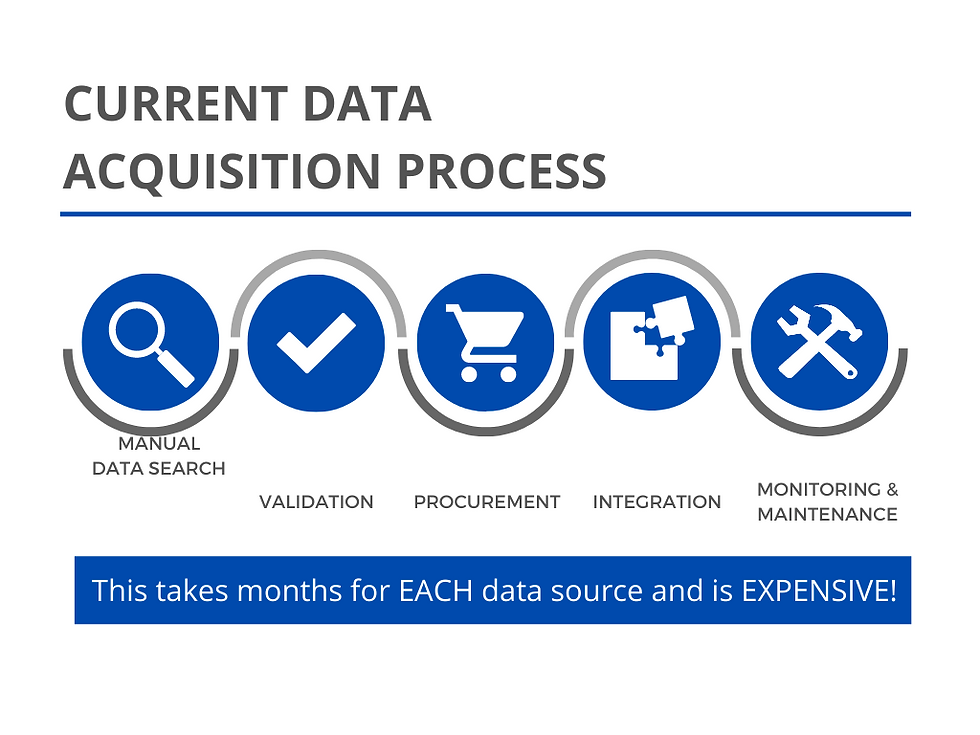
However, nowadays, customers are seeking a better way to do this - in a more automated manner. This is what Explorium is focusing on - automated process and bringing external data under one roof to make the integration of data seamless. Much more creative ways coming from cloud environments, allow you to easily pull the data in, integrate it, transform it, do some matching capabilities along with cleansing the data.
Up until a few years ago, data scientists were spending 85% of their time cleaning the data, preparing it to work on it - even today, data scientists spend 45% of their time in data wrangling. It's necessary to find better processes to make data wrangling less painful so that data scientists can do what they are actually paid for!
With machine learning, data cataloging, and data lakes becoming a part of the stack, it's no longer how it used to be: Data Source → ETL → Data Warehouse → BI. Now we should know which data is to be put where instead of putting it all in one place!
What are some of the technologies to watch this year:
Graph Technology: It helps to refine the search against inventory with context as a way of representing connections based on shopper intent, inside a retailer's data sources. Thus it lets the system build up its internal profile for the customer. Graph databases, graph capabilities within databases for those network types of workloads are some of the growing technologies currently with great potential.
Read more about Graph Technology and Graph Databases
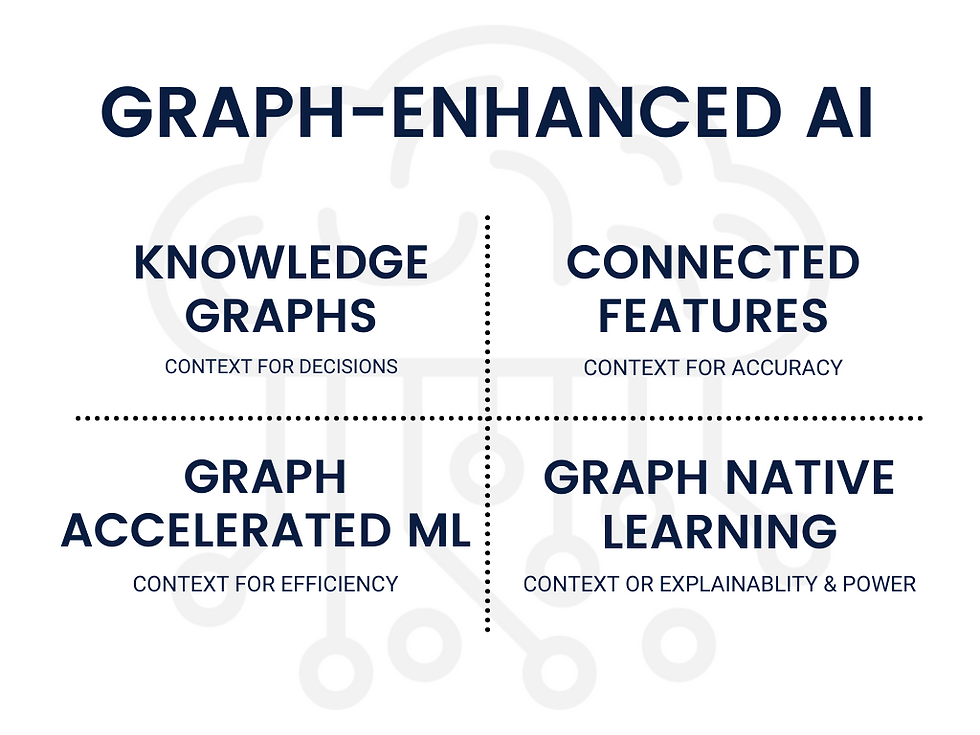
Cloud: This technology has grown exponentially in the recent past and is only going to advance further because of its innumerable benefits.
Stacks: It is not just about the framework used to create models but it extends to your complete data engineering pipeline, business intelligence tools, and how models are deployed. AWS, Azure, Google, and a heterogeneous stack led by Snowflake and Databricks are the top 4 stacks based on popularity.
Data catalogs: It is a collection of metadata, along with data management and search tools, which help analysts and other users of data to find the data that they need. It serves as an inventory of available data and it provides information to evaluate the fitness data for intended uses. We need to make sure that the data catalogs are used proactively instead of reactively.
Read more about Data Catalogs here

Algorithm Library: It defines the functions for various purposes (e.g. searching, sorting, manipulating, counting, etc.) that can operate on a range of elements.
Any technology that can help organizations finding which data is relevant out of all the data that is available to us. In the context of external data, we are seeing more and more organizations trying to use external data and introduce it in their models and BI dashboards.
Data Visualization and its challenges:
Data visualization software companies make it seem like making dashboards is super easy - just get the data and put your dashboard on top of it! But wait, it isn't so easy, unfortunately. There is a lot of work to be done on the data before we can make dashboards on top of it. It is therefore important to educate the consumers about what goes into dashboarding since most of them are only interested in investing in the dashboard or reporting of data that is out there in some shape, mostly a bad shape.
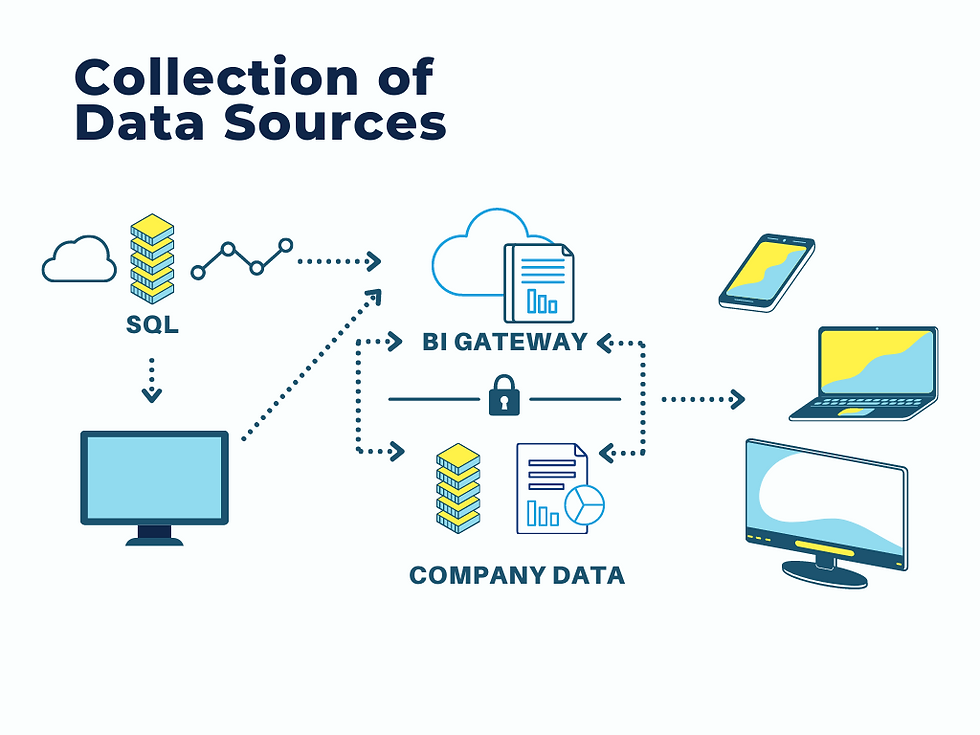
We now need to invest in the infrastructure, architecture, and the quality of data - once that is in place then any BI tool can be used on top of it to get the insights. Certainly, we cannot control everything that's going through the environment, but getting that credibility and having the best tools in the right place where it makes sense goes a long way. If you have good data architecture and good practices in place then you can start having success in the challenging spots.
There is still a need for businesses to understand how important data is to transform the way we work. It's also essential to know how to use this data, both internal and external data collectively to get insights that can assist us to understand our customers better. It's now time to see who can provide the sources in a way that takes into account security and privacy - as that is extremely crucial to organizations.
Comments